This example demonstrates a few ways to specify comparisons and groups in lingmatch.
Built with R 4.4.2 on November 08 2024
Setup
We’ll generate some word category output, in a sort of experimental design that allows for all available comparison types:
Imagine in two studies we paired up participants, then had them have a series of interactions after reading one of a set of prompts:
# load lingmatch
library(lingmatch)
# first, we have simple representations (function word category use frequencies)
# of our prompts (3 prompts per study):
prompts <- data.frame(
study = rep(paste("study", 1:2), each = 3),
prompt = rep(paste("prompt", 1:3), 2),
matrix(rnorm(3 * 2 * 7, 10, 4), 3 * 2, dimnames = list(NULL, names(lma_dict(1:7))))
)
prompts[1:5, 1:8]
#> study prompt ppron ipron article adverb conj prep
#> 1 study 1 prompt 1 4.3998259 2.712729 18.260100 12.171985 17.554020 13.74145
#> 2 study 1 prompt 2 11.0212682 9.010699 3.476042 6.343701 9.610220 10.70595
#> 3 study 1 prompt 3 0.2509456 9.023202 12.049708 11.872618 6.256611 10.97474
#> 4 study 2 prompt 1 9.9777149 8.869178 2.547954 11.451805 9.936199 16.49420
#> 5 study 2 prompt 2 12.4862109 7.785202 7.911950 4.781826 6.692844 10.44815
# then, the same representation of the language the participants produced:
data <- data.frame(
study = sort(sample(paste("study", 1:2), 100, TRUE)),
pair = sort(sample(paste("pair", formatC(1:20, width = 2, flag = 0)), 100, TRUE)),
prompt = sample(paste("prompt", 1:3), 100, TRUE),
speaker = sample(c("a", "b"), 100, TRUE),
matrix(rnorm(100 * 7, 10, 4), 100, dimnames = list(NULL, colnames(prompts)[-(1:2)]))
)
data[1:5, 1:8]
#> study pair prompt speaker ppron ipron article adverb
#> 1 study 1 pair 01 prompt 3 a 5.560151 11.333167 8.750134 12.995852
#> 2 study 1 pair 01 prompt 2 b 10.597623 15.911564 20.095048 1.928010
#> 3 study 1 pair 01 prompt 1 b 8.455429 9.955480 8.321159 3.751488
#> 4 study 1 pair 01 prompt 2 b 9.078023 7.286095 15.348776 12.583830
#> 5 study 1 pair 01 prompt 3 a 11.568424 8.565738 9.107098 11.945809
Matching with a standard
Sample means
Compare each row (here representing a turn in an conversation) with the sample’s mean:
# the `lsm` (Language Style Matching) type specifies the columns to consider,
# and the metric to use (Canberra similarity)
lsm_mean <- lingmatch(data, mean, type = "lsm")
# look at comparison information
lsm_mean[c("comp.type", "comp")]
#> $comp.type
#> [1] "mean"
#>
#> $comp
#> ppron ipron article adverb conj prep auxverb
#> 9.672765 9.902429 10.812763 9.480402 10.038143 9.900312 10.487222
# and maybe the average similarity score
mean(lsm_mean$sim)
#> [1] 0.8344026
This could be considered a baseline for the sample.
Stored means
These LSM categories have some standard means stored internally, as found in the LIWC manual.
# compare with means from a set of tweets
lsm_twitter <- lingmatch(data, "twitter", type = "lsm")
lsm_twitter[c("comp.type", "comp")]
#> $comp.type
#> [1] "twitter"
#>
#> $comp
#> ppron ipron article adverb conj prep auxverb
#> twitter 9.02 4.6 5.58 5.13 4.19 11.88 8.27
mean(lsm_twitter$sim)
#> [1] 0.7287252
# or the means of the set that is most similar to the current set
lsm_auto <- lingmatch(data, "auto", type = "lsm")
lsm_auto[c("comp.type", "comp")]
#> $comp.type
#> [1] "auto: nytimes"
#>
#> $comp
#> ppron ipron article adverb conj prep auxverb
#> nytimes 3.56 3.84 9.08 2.76 4.85 14.27 5.11
mean(lsm_auto$sim)
#> [1] 0.6528981
External means
If you have another set of data, you can also use its means as the comparison:
lsm_prmed <- lingmatch(data, colMeans(prompts[, -(1:2)]), type = "lsm")
lsm_prmed[c("comp.type", "comp")]
#> $comp.type
#> [1] "colMeans(prompts[, -(1:2)])"
#>
#> $comp
#> ppron ipron article adverb conj prep auxverb
#> [1,] 8.788269 8.31949 9.005891 9.92884 9.000049 11.97142 8.663632
mean(lsm_prmed$sim)
#> [1] 0.8240766
Group means
You can also compare to means within groups. Here, studies might be considered groups:
lsm_topics <- lingmatch(data, group = study, type = "lsm")
lsm_topics[c("comp.type", "comp")]
#> $comp.type
#> [1] "study group mean"
#>
#> $comp
#> ppron ipron article adverb conj prep auxverb
#> study 1 10.387083 9.998128 10.89054 9.661167 9.701703 9.631466 10.81388
#> study 2 8.867258 9.794513 10.72506 9.276561 10.417533 10.203479 10.11886
tapply(lsm_topics$sim[, 2], lsm_topics$sim[, 1], mean)
#> study 1 study 2
#> 0.8397258 0.8295869
This type of group variable is just splitting the data, and performing the same comparisons within splits.
Matching with other texts
The previous comparisons were all with standards, where the LSM score could be interpreted as indicating a more or less generic language style (as defined by the comparison and grouping).
Condition ID
Here, prompts constitute our experimental conditions. We have 3 unique prompt IDs, but 6 unique prompts, since each study had its own set, so we need the study and prompt ID to appropriately match prompts:
lsm <- lingmatch(data, prompts, group = c("study", "prompt"), type = "lsm")
lsm$comp.type
#> [1] "prompts"
lsm$comp[, 1:6]
#> ppron ipron article adverb conj prep
#> [1,] 4.3998259 2.712729 18.260100 12.171985 17.554020 13.741453
#> [2,] 11.0212682 9.010699 3.476042 6.343701 9.610220 10.705954
#> [3,] 0.2509456 9.023202 12.049708 11.872618 6.256611 10.974742
#> [4,] 9.9777149 8.869178 2.547954 11.451805 9.936199 16.494196
#> [5,] 12.4862109 7.785202 7.911950 4.781826 6.692844 10.448152
#> [6,] 14.5936464 12.515928 9.789592 12.951105 3.950401 9.464012
lsm$sim[1:10, ]
#> g1 canberra
#> 1 study 1 prompt 3 0.8012121
#> 2 study 1 prompt 2 0.6868380
#> 3 study 1 prompt 1 0.5301802
#> 4 study 1 prompt 2 0.8035201
#> 5 study 1 prompt 3 0.7463066
#> 6 study 1 prompt 1 0.6832114
#> 7 study 1 prompt 3 0.6006250
#> 8 study 1 prompt 2 0.8803764
#> 9 study 1 prompt 3 0.6254127
#> 10 study 1 prompt 1 0.6042073
Here, the group
argument is just pasting together the
included variables, and using the resulting string to identify a single
comparison for each text (acting as a condition ID).
Participant ID
Similarly, participants are only uniquely identified by pair ID and speaker ID (though this could just as well be a single column with unique IDs).
interlsm <- lingmatch(data, group = c("pair", "speaker"), type = "lsm")
interlsm$comp[1:10, ]
#> ppron ipron article adverb conj prep auxverb
#> pair 01 a 8.564288 9.949453 8.928616 12.470831 9.459870 9.933365 10.816552
#> pair 01 b 9.377025 11.051046 14.588328 6.087776 7.930458 12.884964 13.916264
#> pair 02 b 10.620362 7.332231 10.082283 7.130964 7.690392 13.076658 8.714698
#> pair 02 a 14.407274 8.340292 9.359569 7.083569 13.081901 7.773880 13.112972
#> pair 03 a 7.444269 3.900478 10.779339 12.156017 3.872598 11.340337 7.876518
#> pair 03 b 11.320499 8.552962 9.903812 9.912013 7.677445 13.555640 6.019170
#> pair 04 a 11.321233 10.526088 14.155240 16.248495 14.907900 12.206726 8.395467
#> pair 04 b 11.426692 13.527806 7.683133 7.759858 8.772567 4.136878 11.797016
#> pair 05 b 10.285925 8.686241 10.286741 8.083254 8.261563 11.011969 12.032082
#> pair 05 a 8.625059 12.040857 13.744285 8.449484 11.089647 10.483572 10.015284
interlsm$sim[1:10, ]
#> g1 canberra
#> 1 pair 01 a 0.9042582
#> 2 pair 01 b 0.8056024
#> 3 pair 01 b 0.8388374
#> 4 pair 01 b 0.8598558
#> 5 pair 01 a 0.9206056
#> 6 pair 02 b 0.8881149
#> 7 pair 02 a 0.7460497
#> 8 pair 02 b 0.9080004
#> 9 pair 02 a 0.8404195
#> 10 pair 02 b 0.8080563
Matching in sequence
Since participants are having interactions in sequence, we might
compare each turn in sequence. The last entry in the group
argument specifies the speaker:
seqlsm <- lingmatch(data, "seq", group = c("pair", "speaker"), type = "lsm")
seqlsm$sim[1:10, ]
#> group canberra
#> 1 <-> 2, 3, 4 pair 01 0.8172320
#> 2, 3, 4 <-> 5 pair 01 0.8319373
#> 6 <-> 7 pair 02 0.7346971
#> 7 <-> 8 pair 02 0.6880491
#> 8 <-> 9 pair 02 0.7182612
#> 9 <-> 10 pair 02 0.7030160
#> 10 <-> 11 pair 02 0.6786581
#> 12 <-> 13 pair 03 0.8350373
#> 13 <-> 14 pair 03 0.7173501
#> 14 <-> 15 pair 03 0.6857867
The rownames of sim
show the row numbers that are being
compared, with some being aggregated if the same speaker takes multiple
turns in a row. You could also just compare edges by adding
agg = FALSE
:
lingmatch(
data, "seq",
group = c("pair", "speaker"), type = "lsm", agg = FALSE
)$sim[1:10, ]
#> group canberra
#> 1 <-> 2 pair 01 0.6506300
#> 4 <-> 5 pair 01 0.8491029
#> 6 <-> 7 pair 02 0.7346971
#> 7 <-> 8 pair 02 0.6880491
#> 8 <-> 9 pair 02 0.7182612
#> 9 <-> 10 pair 02 0.7030160
#> 10 <-> 11 pair 02 0.6786581
#> 12 <-> 13 pair 03 0.8350373
#> 13 <-> 14 pair 03 0.7173501
#> 14 <-> 15 pair 03 0.6857867
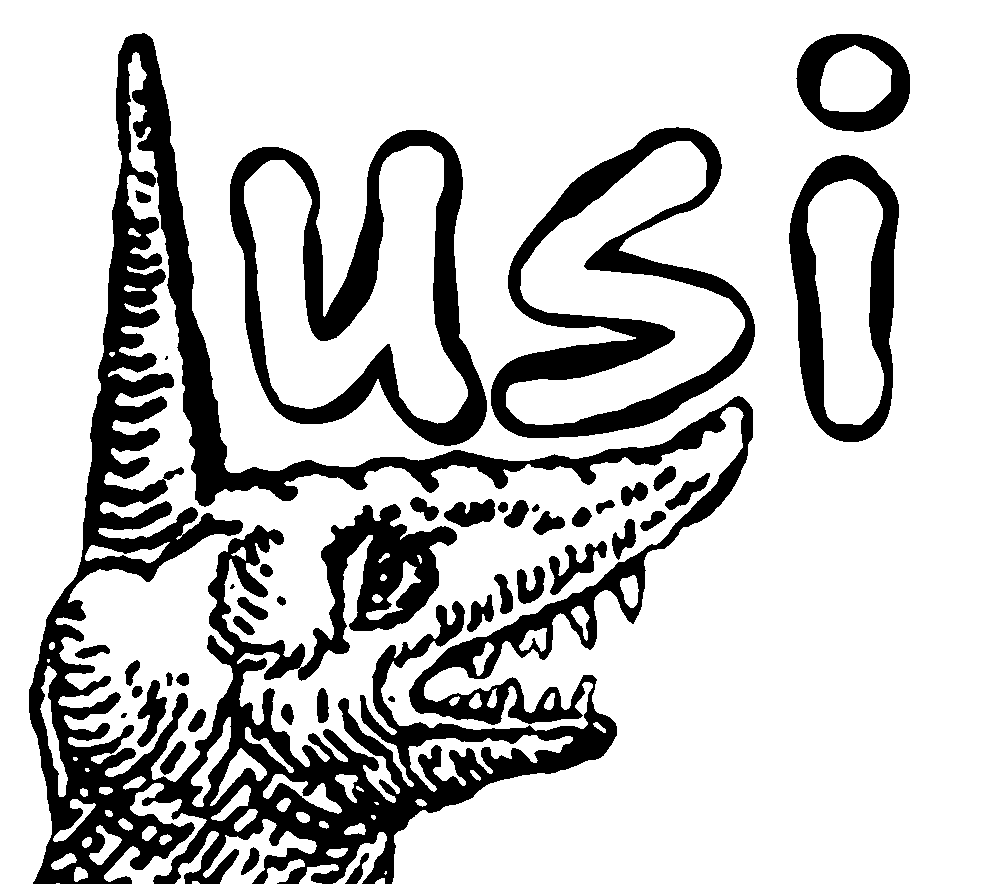